We’re using machine learning to translate tribology test results into real-world performance predictions – an innovative approach that bridges the gap between lab testing and practical applications.
This gives our customers far faster and more cost-effective predictions of real-world performance, compared to extensive and time-consuming physical testing that would otherwise be required.
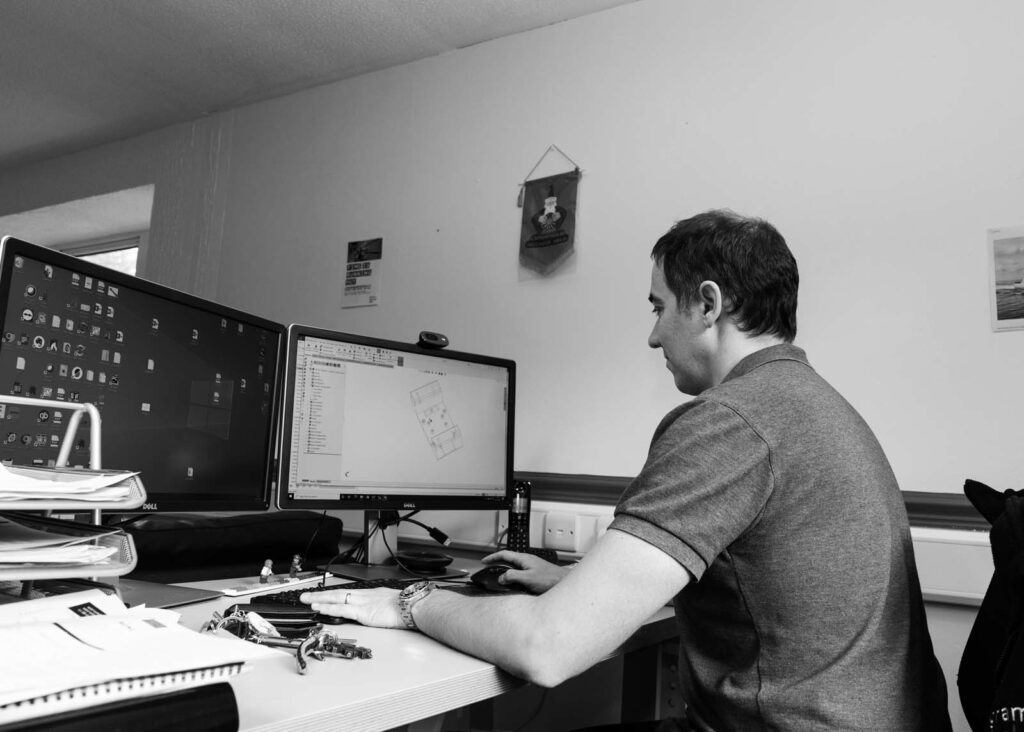
There are a number of steps to deliver an accurate functional model:
- Data Collection: Tribology machines generate copious amounts of data during tests, recording parameters such as friction coefficients, wear rates, temperatures, and more. This data is collected meticulously during controlled lab experiments.
- Data Preparation: To make this data useful for machine learning, it needs to be preprocessed. This involves cleaning, formatting, and organizing the data into a structured dataset. Lab data often undergoes normalization and feature engineering to highlight relevant variables.
- Feature Selection: Not all collected data may be essential for predicting real-world performance. Machine learning models can benefit from feature selection techniques that identify the most relevant variables for the prediction task.
- Model Training: With the prepared dataset and selected features, machine learning models are trained. These models can be various types, such as regression, decision trees, neural networks, or support vector machines. During training, the models learn the relationships between input variables (tribological data) and the target variable (real-world performance).
- Validation: The trained models are validated to ensure their predictive accuracy. This typically involves splitting the dataset into training and validation sets to assess how well the model generalizes to unseen data.
- Model Testing: Once validated, the models can be tested using data collected from tribology machines for new lubricants or materials. These tests simulate real-world conditions as closely as possible.
- Performance Prediction: With the trained and validated machine learning models, it becomes possible to predict how a lubricant or material will perform under specific real-world conditions. For example, the model could predict the wear rate or friction coefficient of a lubricant in an actual engine or industrial machinery, taking into account factors like temperature, load, and speed.
- Continuous Improvement: Machine learning models are not static; they can be continually improved as more real-world data becomes available. The models can adapt and refine their predictions based on the feedback and new information they receive.
The process is complex, but once the model is validated effectively, it facilitates a huge increase in efficiency of real world development and testing.
““Overall good service and a fast expedience of the ordered tests, Ingram Tribology provided excellent assistance on how to best achieve the required objectives for our task. Highly recommended”
Hilmar Danielsen, Senior Researcher, Technical University of Denmark
Tribological Thinking
Find out about our latest thinking, research, news and more.
-
Come and join us…
We are recruiting for a lab technician to come and join our team. As a lab technician at Ingram Tribology you will be working at the cutting edge of lubrication and interfacial engineering sciences, helping to solve problems for companies from all over the world. The deadline for applications is 21st May 2024. To apply,…
-
Gear Micropitting Screening Test
Micropitting is a type of fatigue wear commonly found on gear teeth. The gradual loss of material in the form of small surface pits leads to a change in the geometry of the component; if this is not controlled, it can result in failure of the part. The standardised method of evaluating a lubricants ability…
-
Merry Christmas from all of us at Ingram Tribology
Our Christmas card this year depicts the scene of Santa enjoying the drinks left out for him near the fireplace. Santa is trying both port and hot chocolate and notices a distinct difference in the mouthfeel between the two. The port has a drier, thinner feel. Whilst the hot chocolate impacts a smoother, creamier feeling. …
-
Check out our first Tribo Gatherings Podcast
We have started a Podcast! Our first guest is Dr. Connor Myant from Imperial College. We discuss biotribology, as related to the testing of food and hip joint failures. We discuss the advances in 3D printing and how Connor moved from Tribology into 3D printing. We discuss all Connors current projects and thoughts for the…
-
Preparing for the summer conferences 2023
We have been busy preparing for the conferences this summer. By drafting some of our micropitting work for publication. This will be presented at STLE, Lubmat and TriboUK. We have been busy working on a rapid micropitting test that correlates to the FZG micropitting test. Our new test takes less than 40 hours and correlates…
-
Maintaining safe and quiet railways
We are currently working on an exciting project with the Railway Standards Safety Board (RSSB) and the European Lubricating Grease Institute (ELGI), to develop a new performance test for Top of rail (TOR) materials. These TOR materials are used to ensure traction and reduce noise at the wheel/rail interface. This allows trains to start without…
-
It’s Xmas Penguin Time
This year the animals at the South Pole have been having fun demonstrating the different shapes of contacting bodies.
-
Stribeck Curves
A Stribeck Curve (sometimes also called a Stribeck Friction Curve) is a common name used to described friction vs entrainment speed results, measured on a tribometer. These are very useful as they give a good overview of the frictional performance of a lubricant. The Stribeck Curve is named after the German engineer Richard Stribeck, who…
-
Covid Response 2020
At the beginning of the first COVID lockdown in the UK – March 2020, we like many other people didn’t know what effect the lockdown would have on our business. But we did know and feel like we needed to help our local community. This included the manufacture of protective face visors, using our 3D…